A new paper has been published this week in Optics Express which uses the new, state-of-the-art MAssive GPU cluster for Earth Observation (MAGEO) to retrieve chlorophyll measurements from particulate beam-attenuation coefficients using deep learning.
The research was led by two members of the NEODAAS (NERC Earth Observation Data and Acquisition and Analysis Service) team, including NCEO scientist Giorgio Dall’Olmo based at Plymouth Marine Laboratory.
Particle beam-attenuation coefficient data are measurements of the loss of energy from a beam of light travelling in seawater due to the presence of suspended particles. They are much easier to collect than the current ‘gold standard’ for measuring chlorophyll, which is high-performance liquid chromatography (HPLC). Thus the approach provides a way of collecting significantly more data, which can be used to improve satellite products.
Deep neural networks, algorithms inspired by the human brain, take significant computation resources to train. During initial development the team used a single GPU taking roughly 12 hours per neural network trained. MAGEO allowed the team to accelerate the training of deep neural networks, resulting in nearly 1000 overall trained networks. Thanks to the highly parallel environment and significant individual GPU unit computation power, what would have taken 16 months on a single GPU took 10 days on MAGEO.
The results presented in the study show that the trained neural network can predict chlorophyll to a very high accuracy (-3% bias). Because of the abundance of data, the team were also able to create a wholly independent dataset using the Tara Oceans Expedition. This showed that the neural network could still achieve very high accuracy (-2% bias) in regions and from cruises on which it had not been trained.
“This is a great example of how one can harness the power of deep-learning algorithms to explore existing in-situ datasets and deliver innovative ideas for new satellite-validation instrumentation. The state-of-the-art Massive GPU Cluster for Earth Observation (MAGEO), hosted at the NERC Earth Observation Data Acquisition and Analysis Service (NEODAAS), allowed us to train an ensemble of neural networks that not only provided more accurate results, but also generated estimates of how much we could trust these results.”
Giorgio Dall’Olmo (NCEO scientist at Plymouth Marine Laboratory)
To read more click here
To access the paper click here
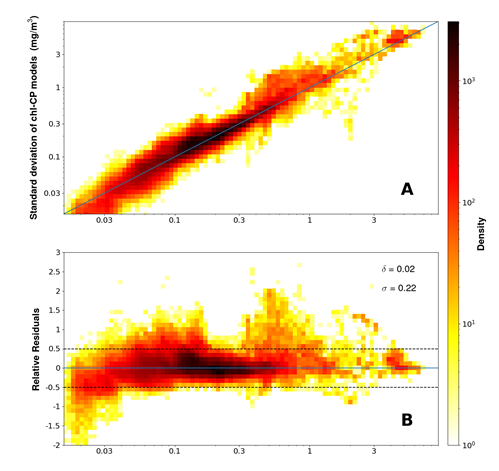