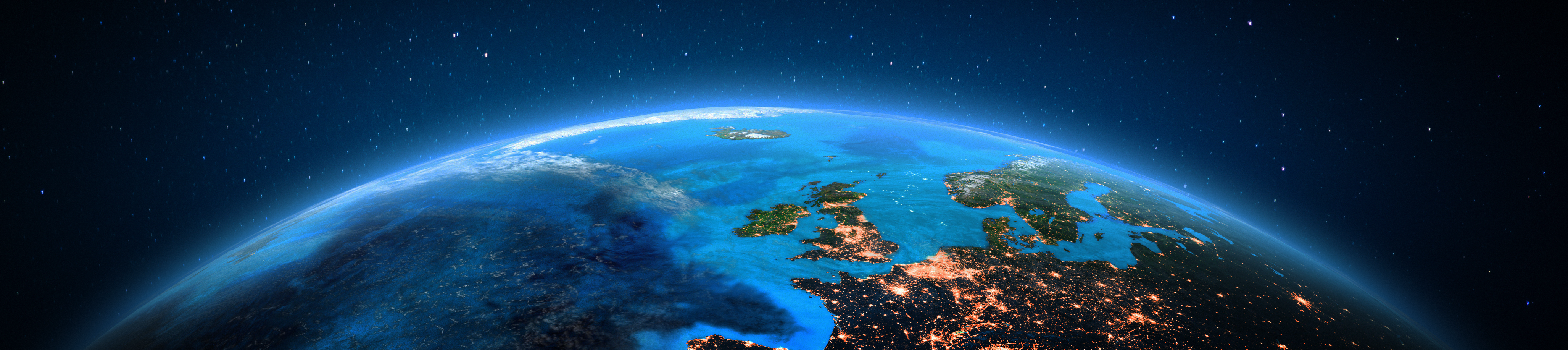
Our People
Dr Yumeng Chen

Data Assimilation Scientific Programmer
Data Assimilation
Research interests
I am interested in the implementation of data assimilation and its applications to climate systems. I work on the parameter estimations in sea ice models, the data assimilation library, parallel data assimilation framework (PDAF), and its application to ocean biogeochemistry models and other fields.
Recent publications
Phytoplankton carbon assimilation in a global ensemble marine ecosystem data assimilation system. 2025-03-18
DOI: https://doi.org/10.5194/egusphere-egu25-3630
Spatiotemporal Variations and Potential Drivers of European Summer Heat Stress. 2025-03-18
DOI: https://doi.org/10.5194/egusphere-egu25-9994
Accurate deep learning-based filtering for chaotic dynamics by identifying instabilities without an ensemble. 2024-09-01
DOI: https://doi.org/10.1063/5.0230837
Tailoring data assimilation to discontinuous Galerkin models. 2024-07
DOI: https://doi.org/10.1002/qj.4737
A Python interface to the Fortran-based Parallel Data Assimilation Framework: pyPDAF v1.0.0. 2024-06-11
DOI: https://doi.org/10.5194/egusphere-2024-1078
Multivariate state and parameter estimation with data assimilation applied to sea-ice models using a Maxwell elasto-brittle rheology. 2024-05-14
DOI: https://doi.org/10.5194/tc-18-2381-2024
DAPPER: Data Assimilation with Python: a Package for Experimental Research. 2024-02-29
DOI: https://doi.org/10.21105/joss.05150
Simplified Kalman smoother and ensemble Kalman smoother for improving reanalyses. 2023-07-27
DOI: https://doi.org/10.5194/gmd-16-4233-2023
Deep learning subgrid-scale parametrisations for short-term forecasting of sea-ice dynamics with a Maxwell elasto-brittle rheology. 2023-07-21
DOI: https://doi.org/10.5194/tc-17-2965-2023
Ensemble Data Assimilation in NEMO using PDAF. 2023-05-15
DOI: https://doi.org/10.5194/egusphere-egu23-3468
Arctic sea ice data assimilation combining an ensemble Kalman filter with a novel Lagrangian sea ice model for the winter 2019–2020. 2023-04-25
DOI: https://doi.org/10.5194/tc-17-1735-2023
Learning and screening of neural networks architectures for sub-grid-scale parametrizations of sea-ice dynamics from idealised twin experiments. 2022-03-27
DOI: https://doi.org/10.5194/egusphere-egu22-5910
Inferring the instability of a dynamical system from the skill of data assimilation exercises. 2021-12-23
DOI: https://doi.org/10.5194/npg-28-633-2021
Extending legacy climate models by adaptive mesh refinement for single-component tracer transport: a case study with ECHAM6-HAMMOZ (ECHAM6.3-HAM2.3-MOZ1.0). 2021-05-03
DOI: https://doi.org/10.5194/gmd-14-2289-2021
Comparison of dimensionally split and multi-dimensional atmospheric transport schemes for long time steps. 2017-10
DOI: https://doi.org/10.1002/qj.3125 ISSN: https://portal.issn.org/resource/ISSN/0035-9009
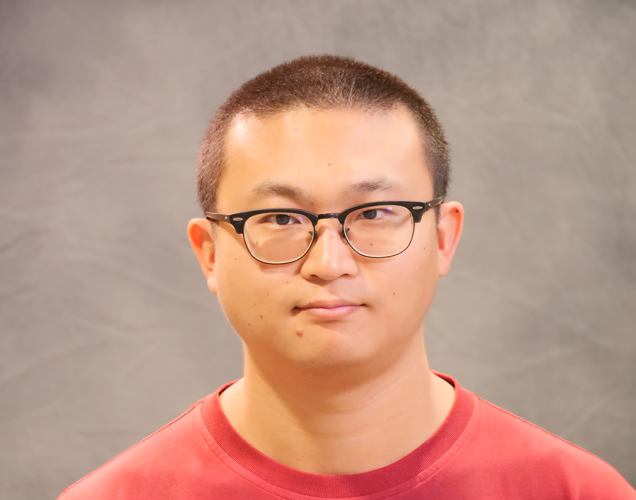